2021 Fall Research Colloquium @ OHS: Neurofencing, Black Holes, and Nanoparticles
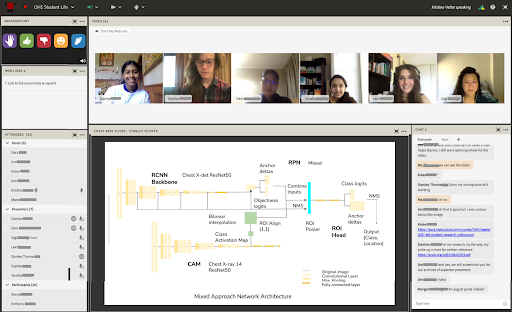
Presenters at the 2021 Stanford OHS Research Colloquium share individual and team research projects.
This year’s series of research colloquia kicked off to an amazing start with seven projects spanning various topics. Read below to find out more about each of these intriguing projects!
Supriya Nair
The first presenter at the colloquium was Supriya Nair, with her project “Applying Neuroscience to Assess Fatigue and Optimize Performance for Young Fencers.” Her project aimed to determine whether a warmup would improve her reaction time during a fencing bout.
During the pandemic, Nair noticed a drop in her reaction time while practicing from home. Nair determined that the only difference between her practices at home and in the club was that she was now skipping/missing/no longer doing a short warm up beforehand. Based on these observations, Nair decided to investigate how much a warm up could improve her reaction time during fencing.
She and her team placed electrodes on the skin and utilized a Backyard Brains SpikerBox in order to measure the spike in muscle activity and, therefore, action potentials used to flex muscles. Based on a stimulus, either visual or auditory, she would lunge forward and make contact with the target, measuring her reaction time and action potential through an EMG each time. She found that just a 15 minute warmup increased her performance by around 15%! Nair also explained that a 15 minute warmup was the optimum warmup as it had the best return on investment.
Finally, Nair had some advice for aspiring student researchers: “Choose something you’re really interested in,” she says, “When you run into problems, your interest in that research is what will keep you going!”.
Alexandra Tabacu
Next up was Alexandra Tabacu, presenting her project “The Future of Diagnosis: Detecting Schizophrenia Using Machine Learning Analysis of EEG Data.” Schizophrenia is a chronic, severe psychotic disorder affecting an estimated 2.6 million adults in the US; current diagnosis relies on qualitative psychiatric evaluation of symptoms by specialists.
Tabacu and her teammates at the Boston University RISE summer program, envisioning a quantitative approach to mental health diagnosis, investigated whether machine learning could be used to identify schizophrenic patients with only their electroencephalogram (EEG) data. EEG tests use electrodes attached to the scalp to record electrical impulses in the brain (‘brain waves’) and are capable of identifying electrical frequency abnormalities associated with schizophrenia.
Using a data set of 84 patients’ EEG readings and nearly 10 million data points, Tabacu and her team created a supervised machine learning algorithm whose most accurate model, RandomForestClassifier, had a True Positive rate of 79.3%- with True Positive signifying the percentage of actual schizophrenic patients who were correctly identified as such.
Tabacu is confident that their machine learning tool will “only become more powerful” due to its potential to improve the efficiency and efficacy of schizophrenia diagnosis.
For more information, see the team’s poster and their project on Github.
Devi Amarsaikhan
Devi Amarsaikhan then presented her project, titled “Sound Waves Reflected by the Auricle.” Originally completed for an IB math assessment, Amarsaikhan investigated the function of the auricle – the visible portion of the external ear – and its effectiveness in amplifying sound waves.
She began by making a clay model of her own ear, then used functions to graph horizontal and vertical ear cross sections and create an approximate 3D model. She then employed 3D vectors in three different modeling methods to calculate the percentage of sound waves coming from different angles that are reflected into the ear canal.
The three mathematical models calculated auricular reflection rates of 50%, 17%, and 56% of sound waves into the ear canal, overall pointing to the effectiveness of the auricle in helping us “appreciate the wondrous sounds of our world.”
For more information, consult Devi’s project writeup.
Gigi Truong
The next presentation was Gigi Truong’s “Self Assembled Lipid Nanoparticles for Drug Delivery in Cancer Treatment.” Truong, while working at Dr. Mu-Ping Nieh’s lab at the University of Connecticut lab over the summer, took part in research on the use of nanoparticles in targeted cancer drug delivery.
Cancer cells hijack the body’s immune system, making it difficult for normal cancer treatments to be implemented effectively. Due to their small size, stability, and flexibility, however, nanoparticles – small particles made of phospholipids (the polar lipids that also make up cell plasma membranes) – can bypass this limitation. Cancerous endothelial tissue exhibits gaps between endothelial cells that are not present in normal tissue; in passive targeting, nanoparticles are able to fit through these endothelial gaps to directly deliver drugs and other treatments.
Truong and her colleagues investigated different morphologies and sizes of nanoparticles – such as differences between three different nanostructures (liposomes, micelles, and bicelles) – to determine the morphologies resulting in highest drug uptake rates by various cancer cells. Truong’s research holds significance for cancer treatment, contributing to the search for less invasive treatment methods.
Tanisha Gupta
The next presenter was Tanisha Gupta, with the project “Mapping Global Poverty Using Computer Vision.” Nearly 35% of the world currently lives in poverty; however, determining impoverished locations using current methods is challenging, which poses problems to effective resource allocation and poverty relief.
Despite 35% of the world currently living in poverty, it is still challenging to effectively allocate resources and alleviate poverty due to difficulties determining impoverished locations with current methods.
Gupta and her team, through the Stanford AI4ALL summer program, investigated the use of artificial intelligence to map low and high poverty areas using satellite imaging, a cheap, easily available, and accurate source of visual information for a region. She and team members developed a logistic regression model by extracting features of the satellite data implicated in poverty determination, such as building density, and testing hyperparameters to determine the best model.
The highest accuracy achieved by the final model was around 60%, an admirable percentage. The model tried to find a linear separation boundary: a straight line could be drawn between ‘poverty’ and ‘no poverty’ when data was graphed. However, this lowered model performance as the data itself was not linearly separable. The researchers aim to use convolutional neural networks, deep learning networks that can recognize objects in visual images, in the future to produce a more effective nonlinear separation boundary.
Damian Musk
Next, Damian Musk presented the project “Behavior of Superconductors of High Critical Temperatures Outside Schwarzschild Black Holes in AdS space”. A Schwarzschild Black Hole is one that has mass, but no electric charge and no spin.
The main goal of this project was to examine condensed matter. Due to strong coupling, condensed matter systems are sometimes difficult to analyze. Musk used the mathematical context of the AdS/CFT correspondence. This mathematical approach, “allows for non-perturbative descriptions in terms of dual weakly coupled systems”.
Musk’s analysis looks at applications of AdS/CFT modeling to holographic condensed matter through modeling a superconductor. This superconductor had a high critical temperature and was outside a Schwarzchild black hole in Anti-de Sitter space. Initially, Musk used a two-dimensional electron condensate Langragian to calculate a free energy analysis and a frequency-dependent conductivity. Musk also examined the asymptotics of both the free energy analysis and frequency-dependent conductivity. Musk then furthered his research using an extended Lagrangian. This one was calculated with a higher-order Maxwell term with a conductivity peak obtained at low frequencies and described well by the Drude model. Musk additionally investigated the “behavior of the Drude model parameter in these limits”.
Stanley Yu
The final presentation at the research colloquium was Stanley Yu’s “Disease Diagnosis and Localization in Chest X-Rays with Mixed Supervision”. Chest Abnormalities are usually diagnosed using chest x-rays which are pretty inexpensive and easy to obtain. However, in order to give a diagnosis to patients these x-Rays must be examined by an expert radiologist.
Yu’s project was aimed at using Artificial Intelligence and more specifically, Machine Learning, to diagnose patients with chest abnormalities. Yu noted that, prior to his research, a similar attempt had been made using solely supervised learning.
Due to the fact that the data available for supervised learning was small, he utilized Mixed Machine Learning to train and test his model. Yu’s model used a large data set with only category labels of normal and abnormal and another much smaller data set with both category and location of diagnosis.
Using this to improve the accuracy of a model with solely supervised learning, Yu was able to significantly increase the accuracy and precision of the diagnosis tool. Yu hopes that mixed supervision models similar to his will be used to make simple, cheap, and quick diagnoses available to everyone around the world. (For more information, Yu’s Slides, Screencast, and Report)
Conclusion:
The 2021 Fall Research Colloquium hosted a variety of informative, enlightening, and inspiring projects by OHS students across a variety of disciplines. For more information about the colloquium, contact Dr. Vetter at [email protected].